I will try to add more information about the concepts behind the tutorial. This way, you will be able to tweak the code and adapt it to your needs. Probably the tutorial code needs some improvements.
Pose estimation (quick theory)
The main concept is pose estimation. You can find some references in the OpenCV doc:
But the main idea is to estimate the transformation between the object frame and the camera frame:
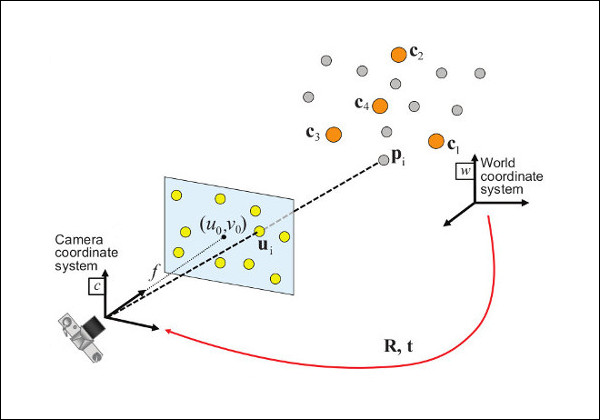
That is:
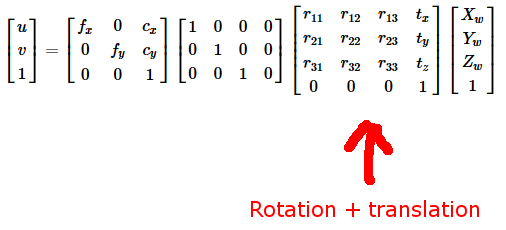
The PnP problem involves to solve for the rotation and the translation that minimize the back-projection error between the set of 3D points expressed in the object frame and the corresponding set of projected 2D image points, assuming a calibrated camera.
Pose estimation (application)
Since camera pose estimation needs a set of 3D object points, we need to find a way to compute these coordinates.
This is what is done in example_tutorial_pnp_registration
:
- perform a training/learning step
- load a mesh file of the desired object, that is simply a CAD model file
- compute the camera pose by manually clicking on some reference points (this is
solvePnP()
) with the 2D/3D correspondences done manually - detect some keypoints on the object and compute the descriptors
- compute the corresponding 3D object points from the 2D image coordinates, the camera pose and the CAD model of the object (see Möller–Trumbore intersection algorithm)
- store in a file the 2D coordinates, the keypoints, the descriptors and the corresponding 3D object coordinates
In example_tutorial_pnp_detection
, the first steps are roughly:
- detect the keypoints in the current image and compute the descriptors
- match the current keypoints with those detected during the training/learning step
- now we have the 2D/3D correspondences and the camera pose can be estimated with
solvePnP()
The tutorial code does also the following things:
- PnP is computed in a robust RANSAC way
- pose is filtered with a Kalman filter (when not enough keypoints are matched to perform
solvePnP()
, the pose can be predicted) - and + some other things
Run the tutorial code
I have observed some issues when the ORB features are detected on multiple levels with solvePnP()
. I don't know why but this can be workaround by using only one pyramid level:
Ptr<Feature2D> orb = ORB::create();
orb.dynamicCast<cv::ORB>()->setNLevels(1);
This should be done both in example_tutorial_pnp_registration
and in example_tutorial_pnp_detection
.
You can extract an image of the video and launch example_tutorial_pnp_registration
on this image to have a new cookies_ORB.yml
file.
If you launch example_tutorial_pnp_detection
with the newly cookies_ORB.yml
file, you should see that it works more or less, depending on the current object view. Indeed, probably when the current object view is too different than the one used during the training/learning step, the matching is not good ... (more)