Finding damages in the image using Feature Matching
Given an image and a template image, I would like to match the images and find possible damages, if any.
Undamaged Image
Damaged Image
Template Image
Note: Above image shows the example of a damage, which can be of any size and shape. Assume that proper preprocessing has been done and both the template and the image are converted to binary with a white background.
I used the following approach to detect the key points and match it:
- Find all the
keypoints
and thedescriptors
from the template as well as the image using ORB. For that, I used the inbuilt function of OpenCV nameddetectAndCompute()
. - After this, I used the Brute Force Matcher and matched it using the
knnMatch()
. - Then I used the
Lowe's Ratio Test
to find good matches.
Results:
If I match the template with itself template-template
, I get 1751 matches which should be an ideal value for a perfect match.
In the undamaged image, I got 847 good matches.
In the damaged image, I got 346 good matches.
We can perceive the differences from the number of matches, but I have a few questions:
- How to pin point the exact location of the damages?
- How can I conclude that the image contains damages by looking at the number of good matches in the
image-template
andtemplate-template
?
Here is the code for your reference.
#include <iostream>
#include <opencv2/features2d/features2d.hpp>
#include <opencv2/calib3d/calib3d.hpp>
#include <opencv2/imgproc/imgproc.hpp>
#include <opencv2/highgui/highgui.hpp>
using namespace std;
using namespace cv;
int main() {
Mat image = imread("./Images/PigeonsDamaged.jpg");
Mat temp = imread("./Templates/Pigeons.bmp");
Mat img_gray, temp_gray;
cvtColor(image, img_gray, CV_RGB2GRAY);
cvtColor(temp, temp_gray, CV_RGB2GRAY);
/**** Pre-processing *****/
threshold(temp_gray, temp_gray, 200, 255, THRESH_BINARY);
adaptiveThreshold(img_gray, img_gray, 255, ADAPTIVE_THRESH_GAUSSIAN_C, THRESH_BINARY_INV, 221, 0);
/*****/
/***** ORB keypoint detector *****/
Mat img_descriptors, temp_descriptors;
vector<KeyPoint> img_keypoints, temp_keypoints;
vector<KeyPoint> &img_kp = img_keypoints;
vector<KeyPoint> &temp_kp = temp_keypoints;
Ptr<ORB> orb = ORB::create(100000, 1.2f, 4, 40, 0, 4, ORB::HARRIS_SCORE, 40, 20);
orb -> detectAndCompute(img_gray, noArray(), img_kp, img_descriptors, false);
orb -> detectAndCompute(temp_gray, noArray(), temp_kp, temp_descriptors, false);
cout << "Temp Keypoints " << temp_kp.size() << endl;
/*****/
vector<vector<DMatch> > featureMatches;
vector<vector<DMatch> > &matches = featureMatches;
Mat & img_desc_ref = img_descriptors;
Mat & temp_desc_ref = temp_descriptors;
BFMatcher bf(NORM_HAMMING2, false); /** Never keep crossCheck true when using knnMatch. Imp: Use NORM_HAMMING2 for WTA_K = 3 or 4 **/
bf.knnMatch(img_descriptors, temp_descriptors, matches, 3);
/*****/
/***** Ratio Test *****/
vector<DMatch> selected;
vector<Point2f> src_pts, temp_pts;
float testRatio = 0.75;
for (int i = 0; i < featureMatches.size(); ++i) {
if (featureMatches[i][0].distance < testRatio * featureMatches[i][1].distance) {
selected.push_back(featureMatches[i][0]);
}
}
cout << "Selected Size: " << selected.size() << endl;
/*****/
/*** Draw the Feature Matches ***/
Mat output;
vector <DMatch> &priorityMatches = selected;
drawMatches(image, img_kp, temp, temp_kp, priorityMatches, output, Scalar(0, 255, 0), Scalar::all(-1));
namedWindow("Output", CV_WINDOW_FREERATIO);
imshow("Output", output);
waitKey();
/******/
return 0;
}
P.S.: I am expecting an elaborate answer as I am new to OpenCV.
feature matching will find the "common" points in your images, but never the differences, imho, you're on the wrong path here.
here's what a simple absdiff -> threshold -> erode -> dilate returns: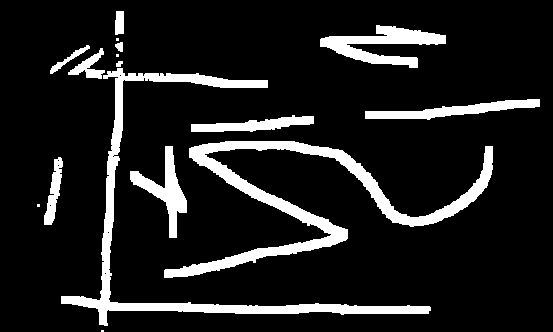
(e.g. you could use above as a mask for inpaint(), to repair the image)
As berak said. Now, if your images aren't perfectly aligned like this one, you will want to use the feature matches to register the images before subtracting. Check THIS tutorial for how to do that.
@berak Thanks for the suggestion. I'll try it out and let you know. @Tetragramm Actually, we are using feature matching to find the keypoints and align the two images. Thanks!
@Tetragramm I tried to align the images using perspectiveTransform but they are still not properly aligned to my liking.